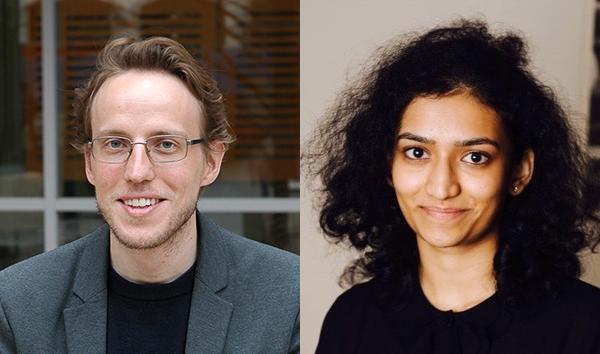
Alex Huth (left), assistant professor of Neuroscience and Computer Science at the University of Texas at Austin. Shailee Jain (right), a Computer Science PhD student at the Huth Lab.
Imagine a world where accessing and interacting with technology doesn’t require keyboard or voice input—just a quick mental command.
Imagine “speech prosthesis” technology that would allow people who are unable to communicate verbally to speak without expensive and highly customized interfaces. Imagine a device that could read a users’ mind, and automatically send a message, open a door, or buy a birthday present for a family member.
This might sound like a distant reality, but research from Alexander Huth and Shailee Jain at Texas Computer Science suggest this future will soon be within reach. Huth and Jain are working toward this technology through research that predicts how the brain responds to language in context.
In a paper presented at the 2018 Conference on Neural Information Processing Systems (NeurIPS), Huth’s team described the results of experiments that used artificial neural networks to predict with greater accuracy than ever before how different parts of the brain respond to specific words.
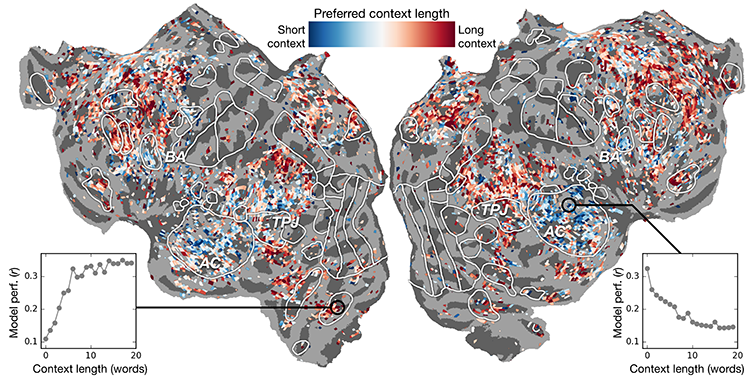
Human perception is complicated, and training computers to imitate naturally occurring processes is challenging. “As words come into our heads, we form ideas of what someone is saying to us, and we want to understand how that comes to us inside the brain,” Huth said. “It seems like there should be systems to it, but practically, that's just not how language works. Like anything in biology, it's very hard to reduce down to a simple set of equations.”
In their work, the researchers ran experiments to test, and ultimately predict, how different areas in the brain would respond when listening to stories. They used data collected from functional magnetic resonance imaging (fMRI) machines, which maps where language concepts are “represented” in the brain.
Using powerful supercomputers at the Texas Advanced Computing Center, they then trained a language model so it could effectively predict what word the participants would think of next—a task akin to Google autocomplete searches, which the human mind is particularly adept at.
“In trying to predict the next word, this model has to implicitly learn all this other stuff about how language works,” said Huth, “like which words tend to follow other words, without ever actually accessing the brain or any data about the brain.”
Based on both the language model and fMRI data, they trained a system that could predict how the brain would respond when it hears each word in a new story for the first time.
Past efforts had shown that it is possible to localize language responses in the brain effectively. However, the new research showed that adding contextual elements—in this case, up to 20 words that came before—improved brain activity predictions significantly. The more context provided, the better the accuracy of their predictions.
Natural language processing—or NLP—has taken great strides in recent years. But when it comes to answering questions, having natural conversations, or analyzing the sentiments in written texts, the field still has a long way to go. The researchers believe their language model can help in these areas.
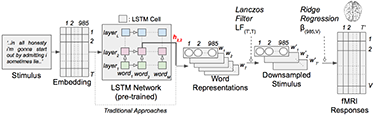
“If this works, then it's possible that this network could learn to read text or intake language similarly to how our brains do,” Huth said. “Imagine Google Translate, but it understands what you're saying, instead of just learning a set of rules.”
With such a system in place, Huth believes it is only a matter of time until a mind-reading system that can translate brain activity into language is feasible.
This system could have life-changing implications for people who aren’t able to communicate verbally. According to Huth, resulting technology could one day provide “speech prosthesis” for people who have lost the ability to speak as a result of injury or disease, as well as people with non-verbal forms of autism.
This technology even might one day translate into a consumer brain-computer interface that could replace keyboard and voice input. “If the technology could be sufficiently miniaturized, we could imagine eventually replacing interfaces that require users to type on a keyboard or speak (like Siri) with direct brain communication” said Huth.
While other labs are also working toward this goal, Huth’s research is especially innovative because his lab is exploring the brain using non-invasive techniques. “Our research uses recording technology like the fMRI, which requires no brain surgery and can be performed on healthy volunteers” said Huth. “While we are more limited in the types of signals we can record, the potential benefit of our approach is larger.”
Regardless of what the future holds, it’s clear that Huth and his team are conducting groundbreaking research that’s both changing our understanding of natural language, and showcasing the power of AI to predict human perception.
This article was adapted from an article recently published on TACC’s website titled “Brain-inspired AI Inspires Insights about the Brain (and Vice-Versa).”
Listen to The College of Natural Sciences' interview with Alex Huth on Point of Discovery: