Original story by Institute for Computational Engineering and Sciences
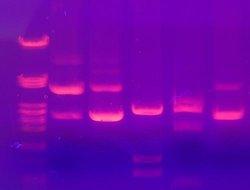
Now, there’s a network for connecting genes and diseases.
Inspired by social networking methods, researchers at The University of Texas’ Institute for Computational Engineering and Sciences (ICES) have developed techniques for discovering connections between genes and the diseases they may influence, like diabetes and Alzheimer’s Disease.
The techniques outperformed all current genetic linkage tests on the market, and uncovered potential new genes of interest for a variety of diseases.
The research results were published in May in the online and open-access journal PLOS ONE.
Common Ground
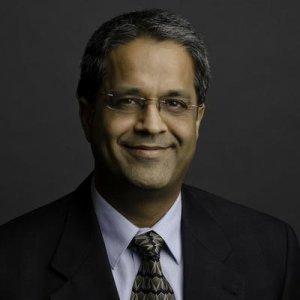
When the two professors met a year and half ago at a presentation, and got to talking, they found that they were working toward the same goal.
“As we started talking we realized that both of us were basically trying to predict missing links,” said Dhillon, who won the the 2013 ICES Distinguished Research Award. “In social networking analysis, it could be between people, or between people and the groups they might belong to or want to join. Whereas, in [Marcotte’s work], the goal is to predict, as of yet, undiscovered links between genes and diseases.”
Dhillon and Marcotte, and their respective research teams, decided to combined forces to create the first “social network” for genes and associated phenotypes, with a focus on finding genes associated with human diseases.
The network is built on a collection of hundreds of thousands of known human gene-to-gene interactions, integrated with thousands of gene-phenotype associations that Marcotte compiled from humans and an array of other organisms, including zebra fish, fruit flies, bacteria, yeast, and even plants.
Although the network is designed to look for human disease genes, including genes from non-human organisms in the network is a smart move because of the shared genetic history between all life. Identifying shared clusters of genes in different species and their phenotypes can help locate potential disease genes in humans, even if those genes have a seemingly unrelated effect in other organisms. Research that Marcotte published in 2010 made this point clear, finding, among other connections, that the same cluster of genes that influence cell wall repair in yeast aids in blood vessel growth in humans. The research was profiled by renowned science writer Carl Zimmer in The New York Times.
The genes that are shared or connected between disparate species and phenotypes is one of the most striking and important features of of Dhillon and Marcotte's network map.
Mice, plants, and people
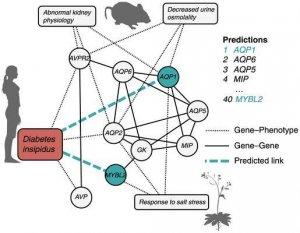
It’s a method akin to Facebook scanning its huge database of users for common interests to come up with a selection of “friend suggestions,” said Dhillon.
A network map included in the research is arranged around diabetes. It’s a web of known connections between genes, genes and phenotypes, and the resulting predicted connections. Included in the network are genes and phenotypes from humans, mice and plants.
“You can think of diabetes like an interest group, and the gene AVPR2 as a person,” said Dhillon, referring to a gene related to kidney function in mice included on the graph.
In this map, amongst known connections across various species, two new gene predictions are highlighted as being potentially important to diabetes based on known connections: AQPI, a gene that influences mouse kidney function, and MYBL2, a gene that influences response to salt stress in plants.
“So the gene AQPI might be linked to diabetes as there are multiple paths [in the gene-phenotype network that suggest this connection.”
From Computers to the Cinic
Diabetes is just one disease that Marcotte and Dhillon investigated with this method in the study. Known gene connections were confirmed, and new potential disease genes found for leukemia, prostate cancer, schizophrenia, and breast cancer, among other conditions.
Dhillon and his research group developed two state-of-the-art machine learning methods to find connections and make gene-phenotype predictions: Katz and Catapult. Both methods identify common genes and associated phenotypes across organisms and rank their likelihood of contributing to a defined disease. But they analyze the data in slightly different ways, with each method having its own networking strengths.
Catapult is good at recovering gene-disease associations that are already known, adding further evidence to support current knowledge. Katz on the other hand, is better at finding associations between diseases and genes that have not been studied well, but make biological sense. For example, a top Alzheimer’s Disease prediction by Katz includes BDKRB2, a gene that is highly expressed in the central nervous system, and associated experimentally with high levels of beta amyloid protein, the main component of plaques often found in the brains of those with Alzheimer’s Disease.
“Both of the algorithm properties can be very useful in different situations. We therefore decided to present both [in the paper],” said Martin Singh-Blom, a former post-doctoral researcher in Marcotte’s lab and lead author in the research paper.
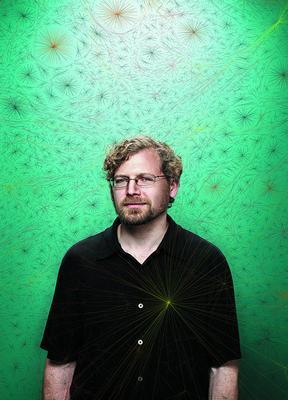
Having a new tool to help tie genes to diseases is invaluable for geneticists, said Singh-Blom, and also for the development of genetic tests used by doctors for serious diseases.
“Angelina Jolie’s recent mastectomy is an example of the benefit this kind of knowledge has given us,” said Singh-Blom, referring to the preventative surgery the actress underwent after genetic tests revealed she carried a variant of the BRCA1 gene strongly associated with breast cancer.
“By improving our knowledge of how mutations in genes cause diseases we can improve our ability to assess the risks and benefits of preemptive treatments like that.”
Article by Monica Kortsha