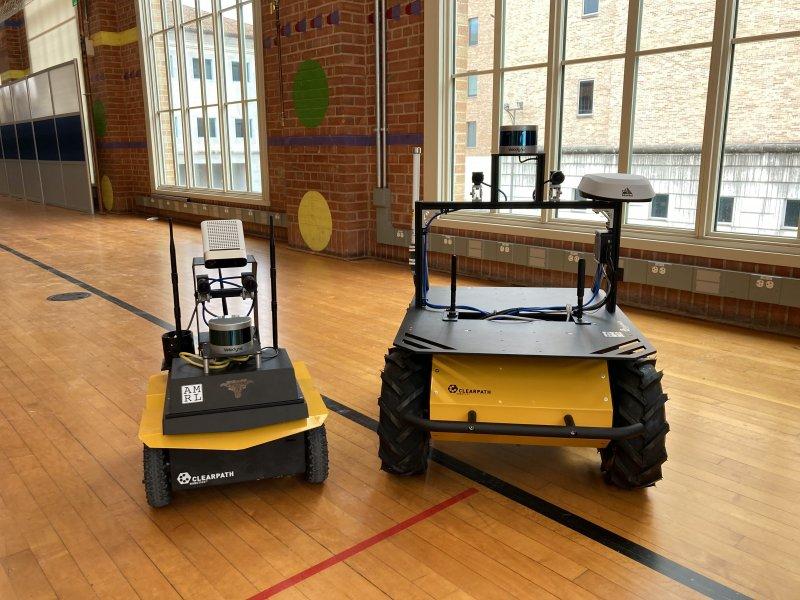
The UT Campus-Jackal (left) and the UT Campus-Husky (right)
A group of Texas Computer Science (TXCS) researchers from the Autonomous Mobile Robotics Laboratory (AMRL) comprising Joydeep Biswas, Sadegh Rabiee, Jarrett Holtz, Kavan Sikand, Max Svetlik, and John Bachman (UMass Amherst) have reached an incredible milestone in their research: deploying an autonomous robot that autonomously navigates on the campus-scale, resilient to everyday changes and varying conditions.
Professor Biswas leads the AMRL, a UT Austin research group that “performs research in robotics to continually make robots more autonomous, accurate, robust, and efficient, in real-world unstructured environments.” In the video above, the robot, referred to as the UT Campus-Jackal, navigated entirely autonomously from The Gates Dell Complex (GDC) to the Anna Hiss Gymnasium (AHG). The route, which was about 0.6 miles, required the robot to reason about its precise state in the world in real-time, despite unexpected variations in the world including construction, vehicular traffic, and pedestrians, stay on-track and avoid going off-course.
Biswas and his team have been working on topics related to long-term outdoor navigation since 2015 when he worked as an assistant professor at The University of Massachusetts at Amherst. The UT Campus-Jackal is one of two platforms (another being a larger robot called UT Campus-Husky) that the team uses to undertake research on perception and planning for long-term autonomy. This topic includes exploration of building perceptual models that can adapt to changing environments in real-time, allowing agents to identify perception failures independently, and learning robot customization from human demonstrations.
A key to the robot’s successful navigation was the localization method used. At the basic level, localization is how an autonomous agent knows where it is on the map, in relation to other elements. The AMRL used episodic non-Markov localization (EnML), a method introduced by Biswas and Manuela M. Veloso, that “reasons about the world as consisting of three classes of objects: long-term features corresponding to permanent mapped objects, short-term features corresponding to unmapped static objects, and dynamic features corresponding to unmapped moving objects.”
Imagine that the robot the AMRL deployed was sent out to navigate autonomously at three different times during UT Austin’s academic calendar: during freshman orientation, during a Student Organization fair, and during midterms. In each scenario, the robot is navigating the same distance and the same path. It can expect that the building and statues that it passes to remain constant throughout each excursion. Yet, each time that it’s deployed, the robot experiences non-static objects that can affect its journey: lost freshmen, tables, packs of students making their way to class. For robots that are deployed long-term, these potential changes need to be accounted for, and the robot has to understand that the map it has of the terrain most likely won’t remain static. EnML is a localization method that helps autonomous agents account for the changes in their environment, to help them navigate in a more natural way.
Biswas stated that the AMRL is “excited to have the robot autonomously perform experiments during such deployments, to improve its own perception algorithms in the wild,” noting that they are “also interested in performing such tasks like mail and package delivery.”
This project is being funded by UT’s Good Systems, which is a grand challenge working at the intersection of artificial intelligence and human values. Read more about these robots and Good Systems’ journey here!